It is well known that the intramuscular fat (IMF) and marbling are both drivers of eating quality in beef and have a positive influence on tenderness, flavour, juiciness and overall taste, which supports its inclusion in carcass grading schemes. Given that the majority of beef carcass classification schemes still largely depend on a visual assessment (such as SEUROP, MSA and AUS-MEAT) and, the laboratory methods for IMF chemical determination are generally destructive and laborious, the beef industry has a significant interest in the development of technologies to improve the precision and objectiveness of the carcass measurements.
That’s why a study was conducted in Australia to test the performance of a prototype vision system in phenotypically different beef carcasses against visual grading of eye muscle area (EMA), MSA and AUS-MEAT marbling score and chemical IMF. The datasets were comprised of Angus, Hereford-Angus cross and Northern Bos indicus cattle breeds. Prior to grading, the carcass images were acquired using a handheld prototype vision camera, which can be seen in the Figure 1 below.
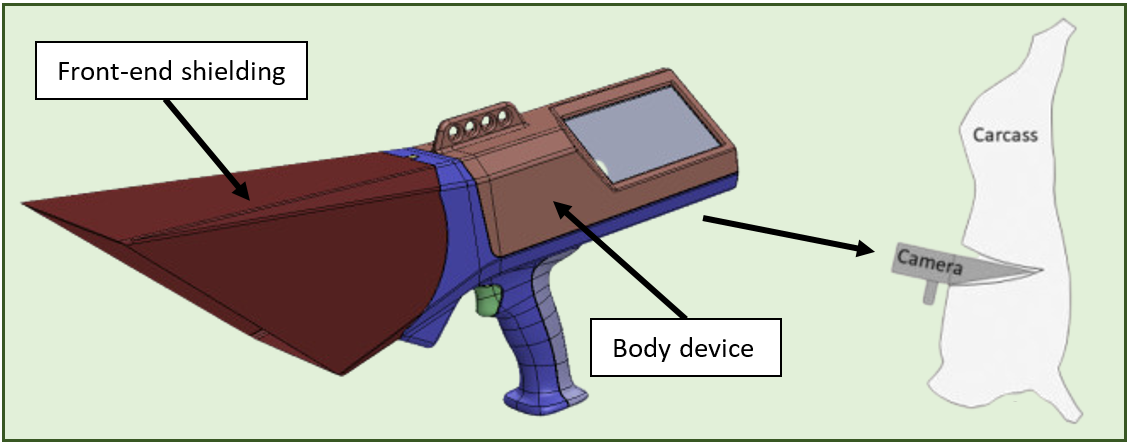
The body device contained the light source, camera, all electronics, a touch display, and an image capture trigger mounted in the handle. The device was connected to a computer by a flexible conduit cable. The carcasses were graded by experienced industry graders accredited in both MSA grading and in AUS-MEAT chiller assessment, and M. longissimus thoracis et lumborum (short loin) was prepared for IMF analysis at the University of New England. The camera shroud was placed over the cut surface to ensure that both left and right eye muscles were captured in the image (Figure 1).
The study demonstrated that the camera prototype for grading beef was able to accurately and precisely predict, in validation, EMA (r2 = 0.83), MSA marbling (r2 = 0.76), AUS-MEAT marbling (r2 = 0.70) and chemical IMF% (r2 = 0.78), however further work to standardise image acquisition considering environmental conditions is required. Further investigations will be undertaken to evaluate the camera system on a wider range of phenotypes for prediction of meat and fat colour.
Marbling is commonly addressed in Australia and USA, supported by consumer studies that showed a strong relationship between beef marbling and consumer satisfaction scores in these countries.
Although in Europe carcass measures such as ribeye area and marbling are not commonly addressed, this type of measurement will probably be more common in the future. In France, at the food conference (“Etats Généraux de l'Alimentation, EGA”), recommendations were made to the meat sector, in particular the introduction of marbling in French beef grading schemes (Liu et al., 2020). Such recommendations perhaps will become a reality in other European countries, and to achieve this purpose, relationships between marbling and European classification scores should be investigated in-depth, and the use of video images analysis could facilitate the process due it is easy to implement in automation systems following the workflow of a slaughterhouse.
Furthermore, similar techniques have been studied for applications in the meat sector for the prediction of several quality parameters and some of these are routinely used in slaughterhouses for carcass grading according to the EU legislation. Examples of these technologies are represented by: Video Image Analysis, X-ray based technologies, Computed Tomography, Dual x-ray absorptiometry and Nuclear Magnetic Resonance.
*Post suggested by Alessandro Ferragina (Teagasc, Ireland).
Authors: Jakeline Vieira Romero, Virginia C. Resconi, Alessandro Ferragina.
Impact on:
![]() | Fast and cheap technique and therefore, easy to implement in automation systems in slaughterhouses. The objective carcass measurements can help to build more trustworthy payment systems. |
![]() | |
![]() | More precise and standardised marbling measurements on the slaughter line. The results can be used for on-farm improvements. |
![]() |
Source of information:
Stewart, S.M. et al. (2021a). Meat Science. 181:108358.
Further information:
- Stewart, S.M. et al. (2021b). Meat Science. 181:108369.
- Liu, J. et al. (2020). Meat Science. 170:108233.
- Craigie, C.R. et al. (2012). Meat Science. 92:307-318.
- Delgado-Pando, G. at al. (2021). Trends in Food Science & Technology. 111:771-782.